AI-Powered Tools and Platforms Revolutionizing Social Science Research
- Tretyak
- 3 days ago
- 5 min read

Artificial Intelligence (AI) is not just a peripheral aid in social science research; it's rapidly becoming the central nervous system, fundamentally transforming the methodologies and capabilities of these disciplines. A new generation of AI-powered tools and platforms is emerging, providing researchers with an algorithmic toolkit of unprecedented power and sophistication, enabling them to explore the human experience with a new level of depth and precision.
I. The Algorithmic Revolution: AI's Arsenal for Social Inquiry
AI-powered tools and platforms are not merely automating existing processes; they are enabling entirely new forms of social science inquiry.
1. Natural Language Processing (NLP) Toolkits: The Algorithmic Decoders of Human Expression
Expanded Description: NLP toolkits provide a comprehensive suite of AI-powered techniques for analyzing, interpreting, and generating human language. They go beyond simple keyword searches, enabling researchers to:
Perform Advanced Sentiment Analysis: Detect subtle emotional nuances, identify shifts in public opinion, and analyze the emotional tone of social interactions.
Conduct Topic Modeling and Discourse Analysis: Uncover the underlying themes, ideologies, and power dynamics within large volumes of text, such as political speeches, news articles, or social media conversations.
Extract Information and Knowledge: Automatically identify and extract key information from unstructured text data, such as names, events, relationships, and arguments.
Examples (Expanded):
Analyzing the evolution of political rhetoric over time using historical text corpora.
Identifying and classifying hate speech or misinformation online.
Summarizing large volumes of qualitative data, such as interview transcripts or ethnographic field notes.
The Algorithmic Power: NLP enables researchers to move beyond qualitative analysis to quantitative and computational approaches to studying language, opening up new avenues for rigorous and large-scale linguistic inquiry.
2. Machine Learning Platforms: The Algorithmic Laboratories of Social Reality
Expanded Description: Machine learning platforms provide a robust and scalable infrastructure for developing, training, deploying, and managing AI models. They offer:
Diverse Algorithm Libraries: A wide range of machine learning algorithms, including supervised, unsupervised, and reinforcement learning methods, tailored for various research tasks.
Automated Model Training and Optimization: Tools for automatically selecting the best algorithms, tuning model parameters, and evaluating model performance.
Distributed Computing Resources: Access to powerful computing resources, including cloud-based infrastructure, enabling researchers to handle massive datasets and complex models.
Examples (Expanded):
Building predictive models to forecast social unrest or political instability based on economic and social indicators.
Developing AI systems to classify individuals into different social groups or categories based on their online behavior or survey responses.
Creating agent-based models to simulate the behavior of complex social systems, such as markets, cities, or organizations.
The Algorithmic Potential: Machine learning platforms empower social scientists to build sophisticated computational models that can capture the complexity and dynamism of social phenomena.
3. AI-Assisted Data Collection and Annotation Tools: The Algorithmic Scribes and Curators
Expanded Description: These tools automate or semi-automate tedious and time-consuming data-related tasks, freeing up researchers to focus on higher-level intellectual work:
Automated Data Extraction: Using AI to automatically extract data from various sources, such as websites, PDFs, or images, reducing manual data entry.
Intelligent Survey Administration: Employing AI-powered chatbots to conduct interactive surveys, adapt to participant responses, and ensure data quality.
AI-Enhanced Data Annotation: Training AI models to automatically label data, such as categorizing text, tagging images, or identifying objects in videos, significantly speeding up the annotation process.
Examples (Expanded):
Using AI to extract data from historical documents, such as census records or legal texts.
Developing AI systems to automatically code and categorize qualitative data, such as interview transcripts or focus group discussions.
Employing AI to track and analyze facial expressions or body language in video recordings of social interactions.
The Algorithmic Efficiency: AI-assisted data collection and annotation tools significantly reduce the time and resources required for data preparation, enabling researchers to conduct larger and more comprehensive studies.
4. Visualization and Interactive Tools: The Algorithmic Storytellers
Expanded Description: AI-powered visualization tools go beyond simple charts and graphs, enabling researchers to create dynamic, interactive, and engaging representations of their findings:
Interactive Data Dashboards: Creating customizable dashboards that allow users to explore data, manipulate variables, and drill down into specific details.
Network Visualization and Analysis: Mapping and visualizing social networks, revealing patterns of relationships, influence, and information flow.
Geospatial Visualization and Mapping: Creating interactive maps that display data across geographic locations, highlighting spatial patterns and relationships.
Virtual Reality and Augmented Reality (VR/AR) Data Exploration: Immersing users in virtual environments to explore data in a more intuitive and engaging way.
Examples (Expanded):
Developing interactive visualizations of social inequality, allowing users to explore how different factors, such as race, gender, and income, intersect.
Creating network visualizations of online communities, showing how information spreads and influence is exerted.
Building virtual reality simulations of historical events, allowing users to experience the past in a more immersive and engaging way.
The Algorithmic Communication: AI-powered visualization tools empower researchers to communicate their findings more effectively to a wider audience, including policymakers, practitioners, and the general public, fostering better understanding and informed decision-making.
II. The Algorithmic Frontier: Ethical Considerations and the Future of Social Inquiry
The hyperdimensional power of AI in social science research necessitates a profound ethical reflection.
Data Privacy and Security: The Algorithmic Oath of Confidentiality
Expanded Description: Protecting the privacy and confidentiality of individuals whose data is used in research is paramount. This includes implementing robust data security measures, anonymization techniques, differential privacy methods, and ensuring compliance with relevant data protection regulations (e.g., GDPR).
Algorithmic Bias and Fairness: The Quest for Algorithmic Justice
Expanded Description: AI algorithms can inadvertently perpetuate or amplify existing social biases if not designed and trained carefully. Researchers must actively work to identify and mitigate biases in their data and algorithms, ensuring that research outcomes are equitable and do not discriminate against any particular group.
Transparency and Explainability: Demystifying the Algorithmic Black Box
Expanded Description: Making AI algorithms more transparent and understandable to researchers and the public is crucial for building trust and ensuring accountability. This involves developing methods for explaining how AI models arrive at their conclusions, providing clear documentation of the data and algorithms used in research, and making AI systems more interpretable.
Informed Consent and Data Ownership: Respecting the Algorithmic Rights of Individuals
Expanded Description: Obtaining informed consent from individuals whose data is used in research is essential, particularly when dealing with sensitive information. Researchers must also consider issues of data ownership and control, ensuring that individuals have a say in how their data is collected, used, and shared, and exploring models of data commons or collective data ownership.
The Social Impact of AI Research: The Algorithmic Responsibility
Expanded Description: Social scientists must carefully consider the potential societal consequences of their AI-driven research. This includes anticipating unintended consequences, addressing potential harms, and ensuring that research findings are used to promote social good and not to exacerbate social inequalities or undermine human rights.
The Algorithmic Renaissance and the Future of Social Understanding
The hyperdimensional integration of AI into social science research represents a renaissance, offering unprecedented opportunities to explore the human condition and address pressing global challenges. However, this renaissance demands a profound commitment to ethical principles, responsible innovation, and a human-centered approach, ensuring that AI empowers social scientists to build a more just, equitable, and informed future.
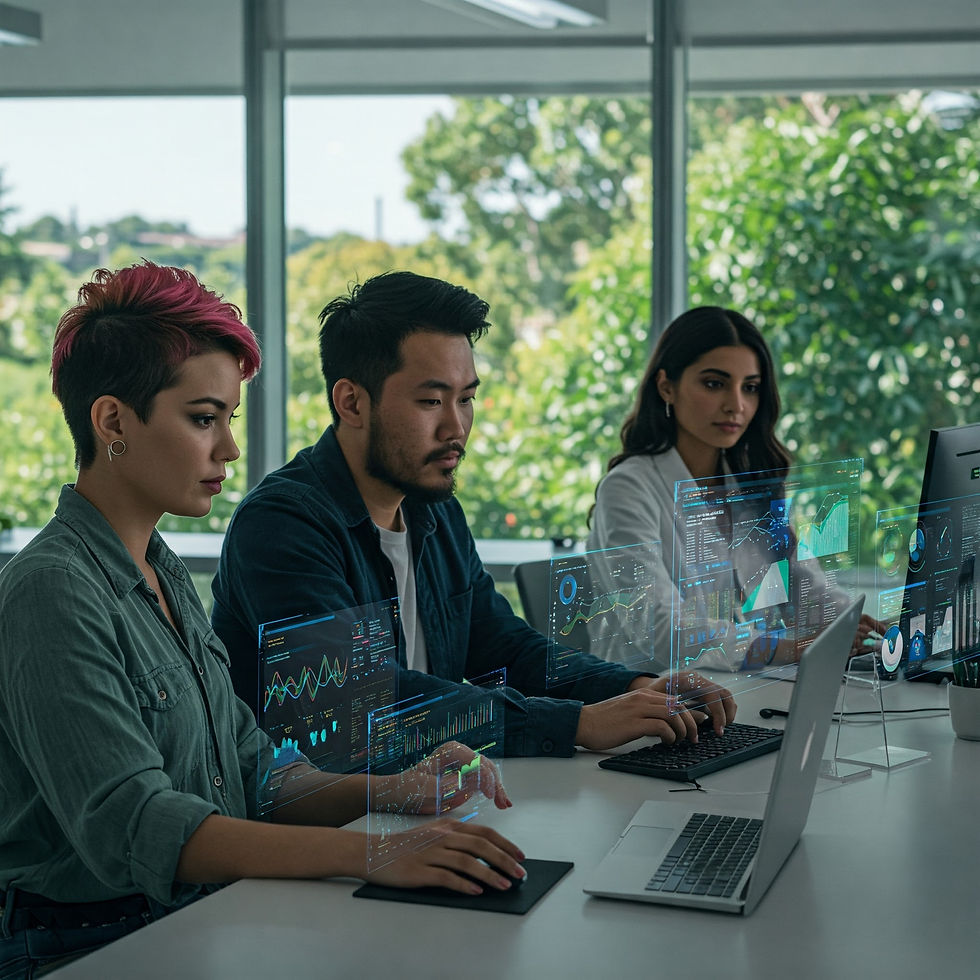
Comments