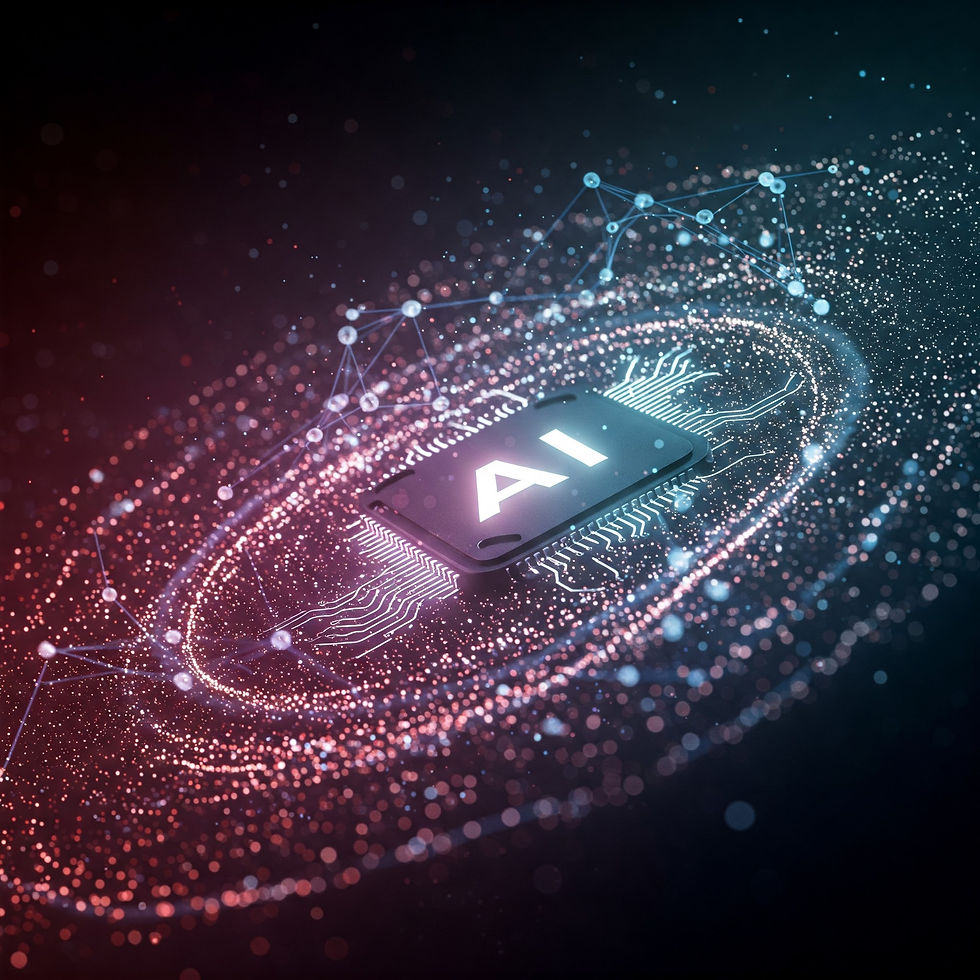
Artificial Intelligence (AI) thrives on data. It's the fuel that powers its learning, adaptation, and ability to perform complex tasks. But the process of acquiring and processing this data is far from simple. AI systems must be able to ingest vast amounts of information from diverse sources, in various formats, and often in real-time. Let's delve deeper into the fascinating world of AI data acquisition and processing, exploring the challenges and opportunities that arise.
Data Acquisition: A Multi-Course Meal for AI
AI systems are not picky eaters. They can consume data from a wide variety of sources, much like a gourmand enjoying a multi-course meal. Here are some of the main ways AI acquires data:
Direct Data Feeds: The Main Course
This is the most common and often the most reliable method of data acquisition. AI systems receive a direct stream of information from various sources, such as:
Sensors: IoT devices, cameras, microphones, and other sensors provide a constant flow of real-time data about the physical world. This is crucial for applications like self-driving cars, robotics, and environmental monitoring.
Databases: AI can access and analyze structured data stored in databases, such as customer information, financial records, and inventory data. This is essential for tasks like customer relationship management, fraud detection, and business intelligence.
APIs: Application Programming Interfaces (APIs) allow AI systems to access data from other applications and services. This enables AI to integrate with various platforms and leverage external data sources. For example, a weather forecasting AI can use APIs to access real-time weather data from meteorological services.
Web Scraping: The Appetizer
AI can be programmed to automatically extract data from websites, online articles, and social media platforms. This is useful for gathering information on a specific topic, tracking trends, or monitoring public opinion. However, web scraping can be challenging due to the constantly changing structure of websites and the need to respect website terms of service.
Example: A market research company uses AI to scrape data from online forums and social media to analyze customer sentiment towards a new product.
Public Datasets: The Side Dish
Many organizations and research institutions make large datasets publicly available for AI research and development. These datasets can contain a variety of data types, including images, text, audio, and video. Using public datasets can be a cost-effective way to train AI models, but it's important to ensure the data is relevant and of high quality.
Example: A research team uses a public dataset of medical images to train an AI model for disease diagnosis.
User Interactions: The Dessert
AI systems can learn from their interactions with users, constantly refining their models and improving their performance. This is particularly important for conversational AI, such as chatbots and virtual assistants.
Example: A customer service chatbot learns from each interaction with a customer, improving its ability to understand natural language and provide relevant responses.
Data Types: A Diverse Menu for AI's Palate
AI systems are capable of processing a wide range of data types, each with its own unique challenges and opportunities.
Text:
Natural language processing (NLP) techniques allow AI to understand and interpret human language, enabling applications like machine translation, sentiment analysis, and text summarization.
Example: An AI-powered legal assistant can analyze legal documents and extract key information, such as clauses and deadlines.
Images:
Computer vision techniques allow AI to "see" and interpret images, enabling applications like object recognition, facial recognition, and image classification.
Example: A security system uses AI to analyze surveillance footage and identify suspicious activity.
Audio:
AI can process audio data to transcribe speech, recognize emotions, and even generate music. This enables applications like voice assistants, speech-to-text software, and music composition tools.
Example: A voice assistant, like Siri or Alexa, uses AI to understand voice commands and respond accordingly.
Video:
AI can analyze video data to understand actions, events, and behaviors, enabling applications like video surveillance, sports analysis, and self-driving cars.
Example: A self-driving car uses AI to analyze video from its cameras to identify pedestrians, other vehicles, and traffic signals.
Code:
AI can understand and even generate code, assisting developers in writing software and automating tasks. This can significantly speed up the software development process and improve code quality.
Example: An AI-powered code completion tool can suggest code snippets and functions to developers, helping them write code more efficiently.
Numerical Data:
AI excels at analyzing numerical data, identifying patterns, and making predictions. This is crucial for applications like financial forecasting, risk assessment, and scientific research.
Example: A financial institution uses AI to analyze market data and predict stock prices.
Real-Time Feast: Handling Information on the Fly
For many AI applications, the ability to process real-time information is crucial. This requires AI systems to be able to ingest and analyze data as it is generated, making decisions and taking actions in a dynamic environment.
Autonomous Systems: Self-driving cars, drones, and robots need to process real-time sensor data to perceive their surroundings, navigate their environment, and make decisions in response to changing conditions.
Example: A drone delivering a package needs to process real-time data from its cameras and sensors to avoid obstacles, adjust its flight path, and ensure safe delivery.
Fraud Detection: AI systems can analyze real-time transaction data to identify and prevent fraudulent activities, such as credit card fraud and identity theft.
Example: A credit card company uses AI to monitor transactions in real-time, flagging suspicious activity and blocking fraudulent transactions.
High-Frequency Trading: AI algorithms can analyze real-time market data to make split-second trading decisions, capitalizing on market fluctuations and maximizing profits.
Example: A hedge fund uses AI to analyze stock market data and execute trades automatically, taking advantage of market opportunities and minimizing risks.
The Challenges of AI's Data Diet
While AI has a voracious appetite for data, there are challenges associated with acquiring and processing information:
Data Quality: The accuracy, completeness, and relevance of data are crucial for training effective AI models. Inaccurate, incomplete, or biased data can lead to poor performance, unreliable results, and even harmful consequences.
Example: An AI model trained on biased data may perpetuate existing societal biases, leading to unfair or discriminatory outcomes.
Data Privacy: AI systems often deal with sensitive information, such as personal data, financial records, and medical histories. Protecting this data from unauthorized access and misuse is essential to maintain trust and comply with regulations.
Example: A healthcare provider using AI to analyze patient data must ensure that the data is anonymized and protected to comply with privacy laws.
Data Bias: AI models can inherit biases from the data they are trained on, leading to unfair or discriminatory outcomes. It's important to address these biases by carefully curating training data and using techniques to mitigate bias in algorithms.
Example: An AI recruitment tool trained on historical hiring data may perpetuate existing gender or racial biases in hiring practices.
The Future of AI Data Consumption
As AI continues to evolve, its ability to acquire and process data will become even more sophisticated. We can expect to see AI systems that can:
Learn from Smaller Datasets: Researchers are developing techniques that allow AI to learn from smaller datasets, reducing the reliance on massive amounts of data for training. This can make AI more accessible and affordable for smaller organizations.
Process Data More Efficiently: Advances in hardware and algorithms are enabling AI to process data more efficiently, improving the speed and accuracy of data analysis. This allows AI to tackle more complex problems and provide faster insights.
Understand and Interpret Data in More Nuanced Ways: AI is becoming increasingly adept at understanding and interpreting data in more nuanced ways, including recognizing emotions, understanding context, and making inferences. This will enable more sophisticated AI applications in fields like customer service, healthcare, and education.
By understanding the complexities of AI's data appetite, we can harness its power to solve complex problems, create innovative solutions, and unlock new possibilities in various fields. As AI continues to evolve, its ability to consume and process information will only become more sophisticated, paving the way for a future where AI plays an even greater role in our lives.
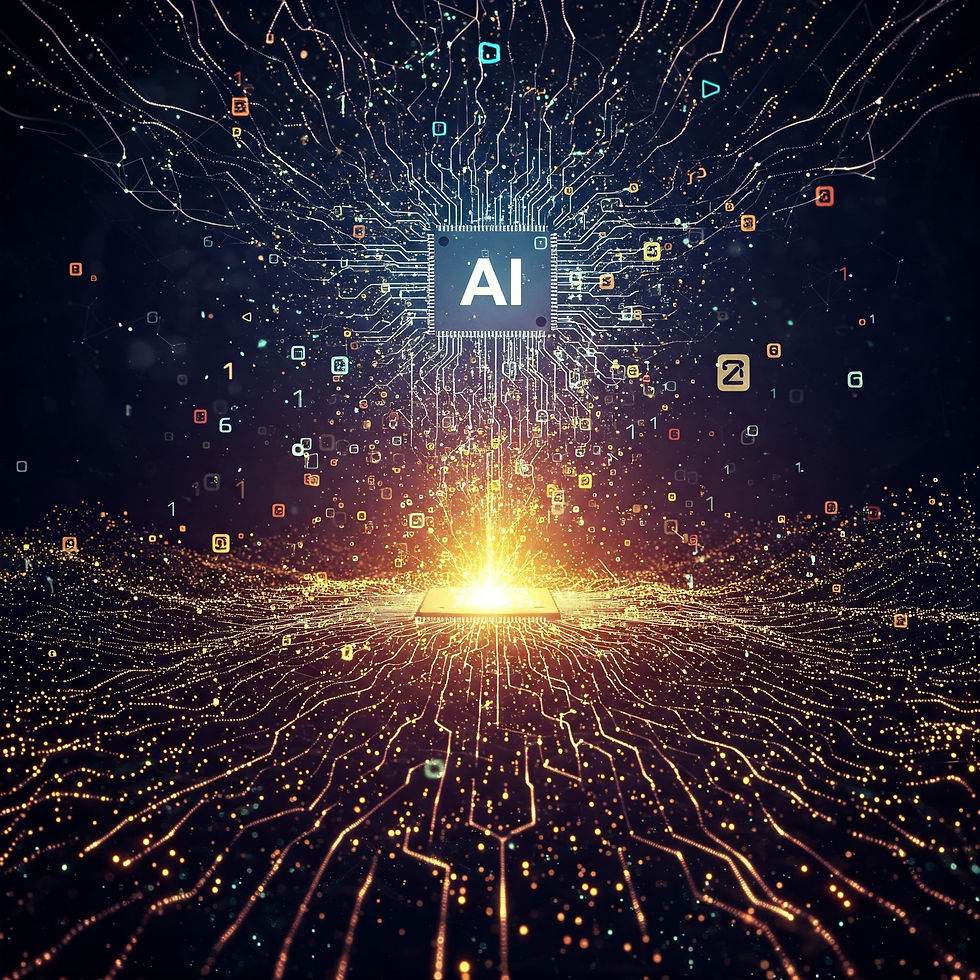
Comments