Ethical Crossroads in AI-Driven Social Science Research
- Tretyak
- 3 days ago
- 5 min read
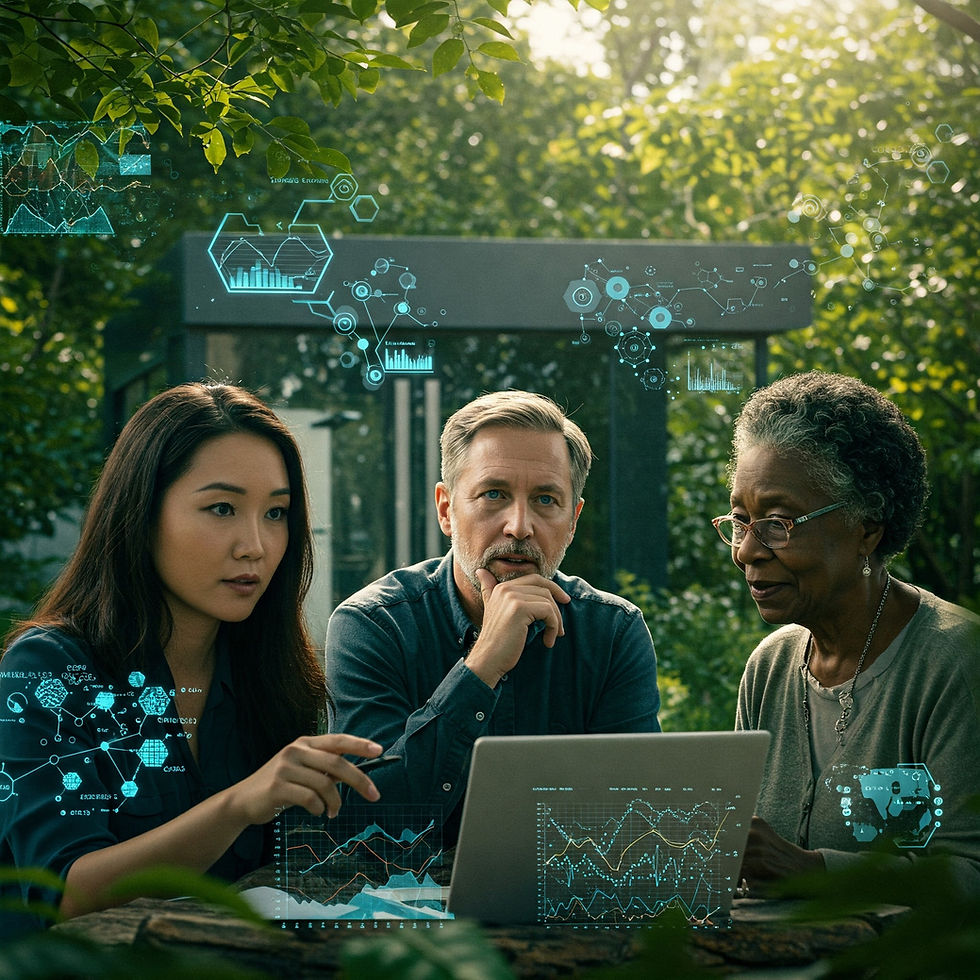
Artificial Intelligence (AI) is rapidly and profoundly transforming social science research, offering powerful new tools to analyze data, model social phenomena, and gain unprecedented insights into human behavior. However, this transformative potential is accompanied by a complex and often perilous labyrinth of ethical considerations that social scientists must navigate with utmost care and responsibility.
I. The Ethical Crossroads: Navigating the Moral Dimensions of AI in Social Research
AI's growing presence in social science research raises a host of ethical challenges that require careful scrutiny and proactive solutions.
1. Data Privacy and Security: Safeguarding the Algorithmic Rights of Individuals
Expanded Description: Social science research often involves the collection and analysis of sensitive data about individuals, including:
Personal identifiable information (PII): Names, addresses, contact information.
Demographic data: Age, gender, ethnicity, socioeconomic status.
Behavioral data: Online activity, purchasing habits, social interactions.
Beliefs and attitudes: Political opinions, religious views, cultural values.
AI algorithms, with their capacity for large-scale data processing and pattern recognition, can potentially expose or misuse this data, leading to:
Privacy breaches: Unauthorized access to or disclosure of personal information.
Surveillance: Tracking and monitoring individuals' activities without their knowledge or consent.
Discrimination: Using data to make decisions that unfairly disadvantage certain groups.
Expanded Ethical Considerations:
Anonymization and De-identification: Employing robust techniques to remove or obscure identifying information from data, while still preserving its analytical value.
Differential Privacy: Adding statistical noise to datasets to protect individual privacy, while allowing for aggregate analysis.
Secure Data Storage and Access Control: Implementing stringent security measures to protect data from unauthorized access, use, or modification.
Data Minimization: Collecting only the data that is strictly necessary for the research purpose.
Informed Consent and Transparency: Obtaining explicit and informed consent from participants about how their data will be collected, used, shared, and stored, and being transparent about the AI algorithms used in the research.
Example: Analyzing social media data to study online harassment necessitates rigorous anonymization techniques to prevent the identification and victimization of individuals.
2. Algorithmic Bias and Fairness: Ensuring Equitable and Just Outcomes
Expanded Description: AI algorithms are trained on data, and if that data reflects existing social inequalities and prejudices, the algorithms can perpetuate or even amplify those biases, leading to:
Representation bias: Certain groups being underrepresented or misrepresented in the training data.
Historical bias: The training data reflecting past social injustices.
Measurement bias: Flawed or biased ways of measuring social phenomena.
This can result in AI models that:
Produce inaccurate or unreliable results for certain groups.
Make decisions that unfairly disadvantage individuals or communities.
Reinforce existing social hierarchies and power structures.
Expanded Ethical Considerations:
Bias Detection and Mitigation: Developing methods to identify and remove biases from training data and AI algorithms, including techniques for data augmentation, re-sampling, and adversarial debiasing.
Fairness Metrics and Evaluation: Defining and using appropriate metrics to assess the fairness of AI models, such as equal opportunity, equal outcome, and counterfactual fairness.
Algorithmic Auditing and Accountability: Implementing mechanisms for auditing AI algorithms and holding developers and researchers accountable for their fairness and impact.
Diversity in AI Development Teams: Promoting diversity among AI developers and researchers to ensure a broader range of perspectives and values are considered.
Example: AI models used to predict criminal recidivism or loan risk may exhibit racial bias, leading to discriminatory outcomes in the criminal justice system or financial lending.
3. Transparency and Explainability: Demystifying the Algorithmic Black Box
Expanded Description: Many AI algorithms, particularly deep learning models, operate as "black boxes," meaning that their decision-making processes are opaque and difficult for humans to understand. This lack of transparency raises concerns about:
Trust: Eroding trust in AI-driven research findings.
Validity: Making it difficult to validate the accuracy and reliability of AI models.
Accountability: Hindering the ability to identify and correct errors or biases in AI systems.
Expanded Ethical Considerations:
Explainable AI (XAI): Developing methods to make AI algorithms more transparent, interpretable, and understandable to researchers and the public, such as techniques for feature importance analysis, rule extraction, and counterfactual explanation.
Model Validation and Robustness Testing: Rigorously validating AI models using diverse datasets and stress-testing them to identify potential weaknesses or vulnerabilities.
Documentation and Reproducibility: Providing clear and comprehensive documentation of the data, algorithms, and methodologies used in AI-driven research to ensure reproducibility and facilitate scrutiny by other researchers.
Example: An AI model that predicts public opinion based on social media data may be difficult to interpret if it doesn't provide insights into which specific words or phrases influenced its predictions.
4. The Potential for Misuse: Responsible Innovation and Ethical Governance
Expanded Description: AI tools, while offering immense benefits, can also be misused for unethical or harmful purposes, necessitating careful consideration of potential risks and the development of robust governance mechanisms.
Potential Misuses:
Surveillance and Social Control: AI can be used to monitor and track individuals' behavior, potentially infringing on civil liberties and privacy rights.
Manipulation and Propaganda: AI can generate highly persuasive and targeted propaganda or disinformation, undermining democratic processes.
Automation of Bias and Discrimination: AI can automate and scale discriminatory practices, perpetuating existing inequalities in society.
Expanded Ethical Considerations:
Dual-Use Technology Awareness: Recognizing that AI technologies can have both beneficial and harmful applications and anticipating potential misuse scenarios.
Ethical Guidelines and Codes of Conduct: Developing and implementing ethical guidelines and codes of conduct for AI development and deployment in social science research.
Public Engagement and Education: Engaging in open and informed discussions with the public about the ethical implications of AI and fostering media literacy to combat misinformation.
Regulatory Frameworks and Oversight Mechanisms: Establishing appropriate regulatory frameworks and oversight mechanisms to govern the development and use of AI, ensuring accountability and preventing misuse.
Example: AI tools used to analyze facial expressions or body language could be misused for surveillance or discriminatory profiling.
5. The Philosophical and Societal Impact: Re-evaluating the Human Condition in the Algorithmic Age
Expanded Description: AI's increasing role in social science research raises fundamental questions about the nature of human agency, social structures, and the future of society.
Philosophical and Societal Implications:
The Re-definition of Human Agency: How does AI influence our understanding of free will, decision-making, and individual responsibility?
The Transformation of Social Structures: How will AI reshape social institutions, such as education, work, and governance?
The Future of Human-AI Interaction: How will humans and AI interact and coexist in the future, and what are the implications for social relationships and cultural values?
Expanded Ethical Considerations:
Interdisciplinary Dialogue: Fostering collaboration between social scientists, philosophers, ethicists, and technologists to address these complex questions.
Critical Reflexivity: Encouraging social scientists to critically examine their own assumptions and biases in the context of AI-driven research.
Public Discourse and Policy Recommendations: Engaging in public discourse and providing evidence-based recommendations to policymakers about the ethical and societal implications of AI.
III. The Quantum Path Forward: Towards an Ethical and Human-Centered Algorithmic Social Science
Navigating the ethical labyrinth of AI in social science research requires a fundamental commitment to responsible innovation, ethical governance, and a human-centered approach.
Embracing a Holistic Ethical Framework: Moving beyond a narrow focus on technical solutions to embrace a broader ethical framework that encompasses values such as justice, fairness, respect for human dignity, and social responsibility.
Fostering Interdisciplinary Collaboration: Cultivating collaboration among social scientists, computer scientists, ethicists, policymakers, and community stakeholders to ensure that AI language technologies are developed and deployed responsibly.
Promoting Data Justice and Algorithmic Equity: Prioritizing data diversity, quality, transparency, and ethical data collection practices to mitigate bias and promote fairness in AI algorithms.
Cultivating Algorithmic Literacy and Public Awareness: Educating the public about the capabilities and limitations of AI, empowering them to critically evaluate AI-driven information and participate in informed decision-making.
Shaping the Future of Social Science Research: Actively engaging in shaping the future of AI research and development, ensuring that it aligns with ethical principles and promotes the well-being of individuals and society.
The journey into this new era of AI-driven social science research is both exciting and fraught with peril. By embracing ethical vigilance, fostering interdisciplinary collaboration, and prioritizing human values, we can harness the transformative potential of AI to create a future where knowledge empowers us to build a more just, equitable, and flourishing society.
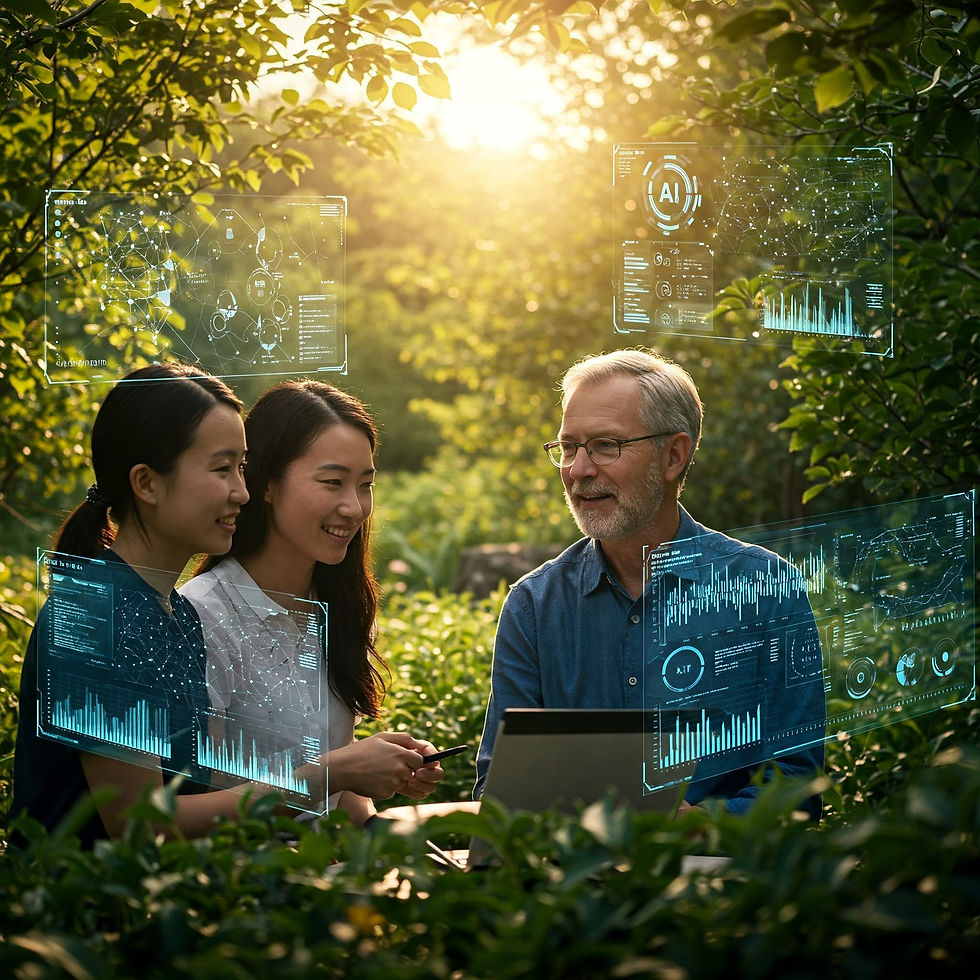
Comments