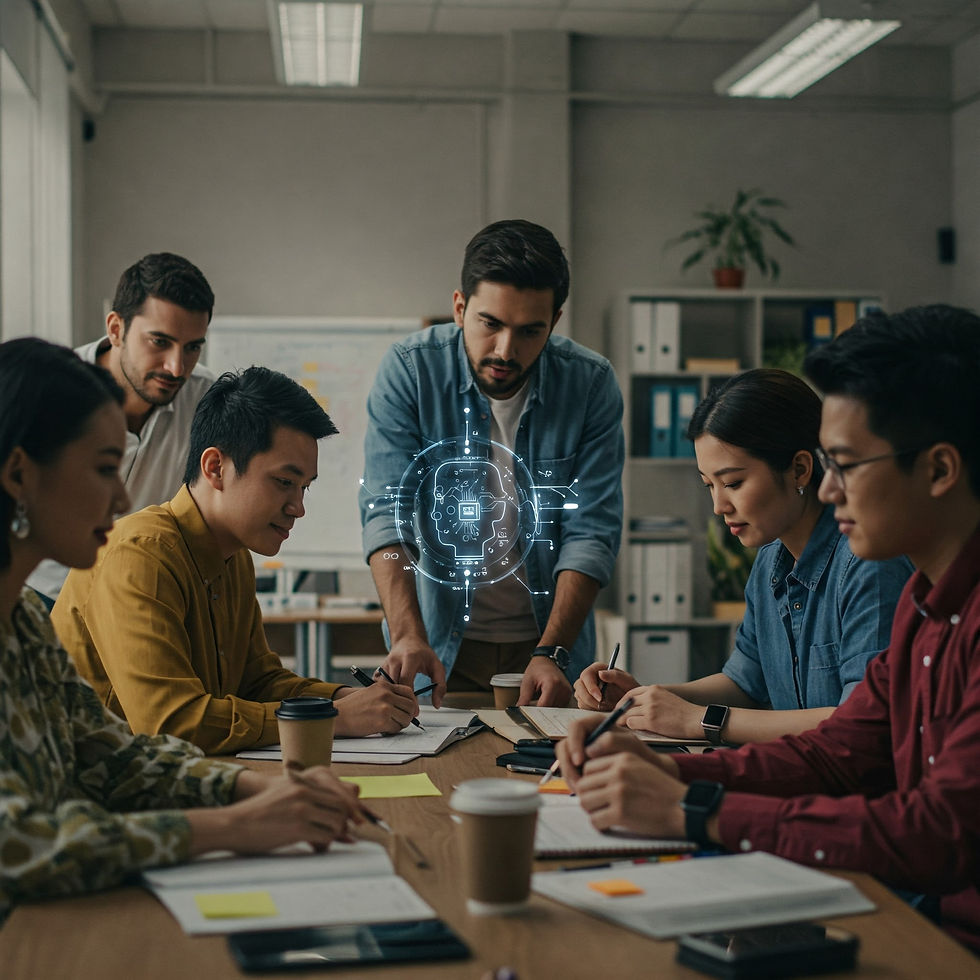
Artificial Intelligence (AI) has the potential to revolutionize many aspects of our lives, but it also carries the risk of perpetuating and even amplifying existing societal biases. From biased datasets to discriminatory algorithms, the development and use of AI raise critical questions about fairness, equity, and justice. How can we ensure that AI systems are free from bias and promote a more just and equitable society?
The Roots of Bias in AI: Unmasking the Hidden Prejudices
Bias in AI can be insidious, creeping into systems through various channels:
Biased Data: The Perils of Prejudice
AI systems learn from vast amounts of data, and if this data reflects existing societal biases, the AI will likely inherit those biases. This can lead to discriminatory outcomes, perpetuating and even amplifying existing inequalities.
For example, if a facial recognition system is trained on a dataset that predominantly features white faces, it may be less accurate at recognizing people of color, leading to misidentification, false arrests, and other harmful consequences. Similarly, a hiring algorithm trained on historical data that reflects gender bias in hiring practices may unfairly disadvantage female candidates.
Algorithmic Bias: The Hidden Hand of Code
Even with unbiased data, the algorithms themselves can introduce bias. This can occur due to the way the algorithm is designed, the assumptions it makes, or the way it interacts with other components of the AI system.
For example, an algorithm designed to predict recidivism (the likelihood of a criminal reoffending) may inadvertently perpetuate racial bias if it relies on factors that are correlated with race, such as socioeconomic status or neighborhood crime rates. This could lead to harsher sentences or unfair treatment for individuals from certain racial groups.
Human Bias: The Unconscious Influence
The developers who create AI systems can also introduce their own biases, consciously or unconsciously. This can influence the choices they make about the data, the algorithms, and the objectives of the AI system.
For example, a developer who holds unconscious biases about gender roles may inadvertently design an AI system that reinforces those biases, such as a virtual assistant that is programmed to be more subservient when interacting with male users.
The Consequences of Bias in AI: Real-World Harm
Bias in AI can have serious consequences, impacting individuals and society as a whole:
Discrimination: Perpetuating Inequality
Biased AI systems can discriminate against certain groups, denying them opportunities, resources, or fair treatment. This can perpetuate existing inequalities and create new forms of injustice, undermining social progress and eroding trust in institutions.
For example, a biased loan application system could unfairly deny loans to individuals from certain racial or ethnic groups, hindering their ability to buy homes, start businesses, or access education.
Inaccuracy: Errors with Real-World Consequences
Biased AI systems can be less accurate for certain groups, leading to errors, misclassifications, and unfair outcomes. This can have serious consequences in areas like healthcare, criminal justice, and education.
For example, a biased medical diagnosis system could misdiagnose or mistreat patients from certain groups, leading to delayed treatment, worsened health outcomes, or even death.
Lack of Trust: Eroding Confidence in AI
Bias in AI can erode trust in these systems, making people less likely to use or adopt them. This can hinder the potential benefits of AI and slow down its adoption in various fields.
For example, if people believe that facial recognition systems are biased against certain racial groups, they may be less likely to support their use in law enforcement or other public settings.
Building Fair and Equitable AI: A Multi-Pronged Approach
To prevent AI systems from perpetuating or amplifying existing societal biases, we need to adopt a multi-faceted approach that addresses the root causes of bias and promotes fairness and equity throughout the AI lifecycle:
Diverse and Representative Data: Reflecting the Real World
Ensure that the data used to train AI systems is diverse and representative of the population, including all relevant demographics and groups. This can help mitigate bias and ensure that AI systems are fair and accurate for everyone.
This requires careful data collection and curation, as well as techniques like data augmentation to address imbalances and underrepresentation in existing datasets.
Fairness-Aware Algorithms: Designing for Equity
Develop algorithms that are explicitly designed to be fair and unbiased. This can involve incorporating fairness constraints into the algorithm design, using techniques like adversarial debiasing, or developing new fairness metrics.
Fairness-aware algorithms aim to ensure that AI systems do not discriminate against certain groups, even if the data contains biases. This requires careful consideration of different notions of fairness and the potential trade-offs between different fairness criteria.
Transparency and Explainability: Opening the Black Box
Make AI systems more transparent and explainable, allowing humans to understand how they work and identify potential biases. This can involve techniques like visualizing decision trees, highlighting important features, or generating natural language explanations.
Transparency and explainability are crucial for building trust in AI systems and ensuring that they are used responsibly and ethically. They also allow for scrutiny and accountability, enabling humans to identify and correct biases or errors in AI systems.
Human Oversight and Accountability: Keeping Humans in the Loop
Ensure that humans are involved in the development and deployment of AI systems, and that there are clear lines of responsibility for AI decisions. This can help prevent unintended consequences and ensure that AI systems are used ethically and responsibly.
Human oversight can involve various mechanisms, such as human-in-the-loop systems, where humans can intervene or override AI decisions, or ethical review boards that assess the ethical implications of AI systems before they are deployed.
Ethical Review and Auditing: Ensuring Compliance
Conduct regular ethical reviews and audits of AI systems to identify and address potential biases. This can involve independent experts reviewing the AI system, its data, and its algorithms to ensure that it meets ethical standards and fairness criteria.
Ethical review and auditing can help ensure that AI systems are developed and used in a way that aligns with human values and promotes fairness and equity. It can also help identify and address potential biases or ethical concerns before they lead to harm.
The Path Forward: Towards a More Just and Equitable AI
Building fair and equitable AI is an ongoing challenge that requires collaboration, innovation, and a commitment to ethical principles. By addressing the roots of bias in AI and adopting proactive measures to mitigate its impact, we can harness the power of AI to create a more just and equitable society for all. This requires a collective effort from researchers, developers, policymakers, and the public to ensure that AI is developed and used in a way that benefits everyone, regardless of their background or identity.

Commenti