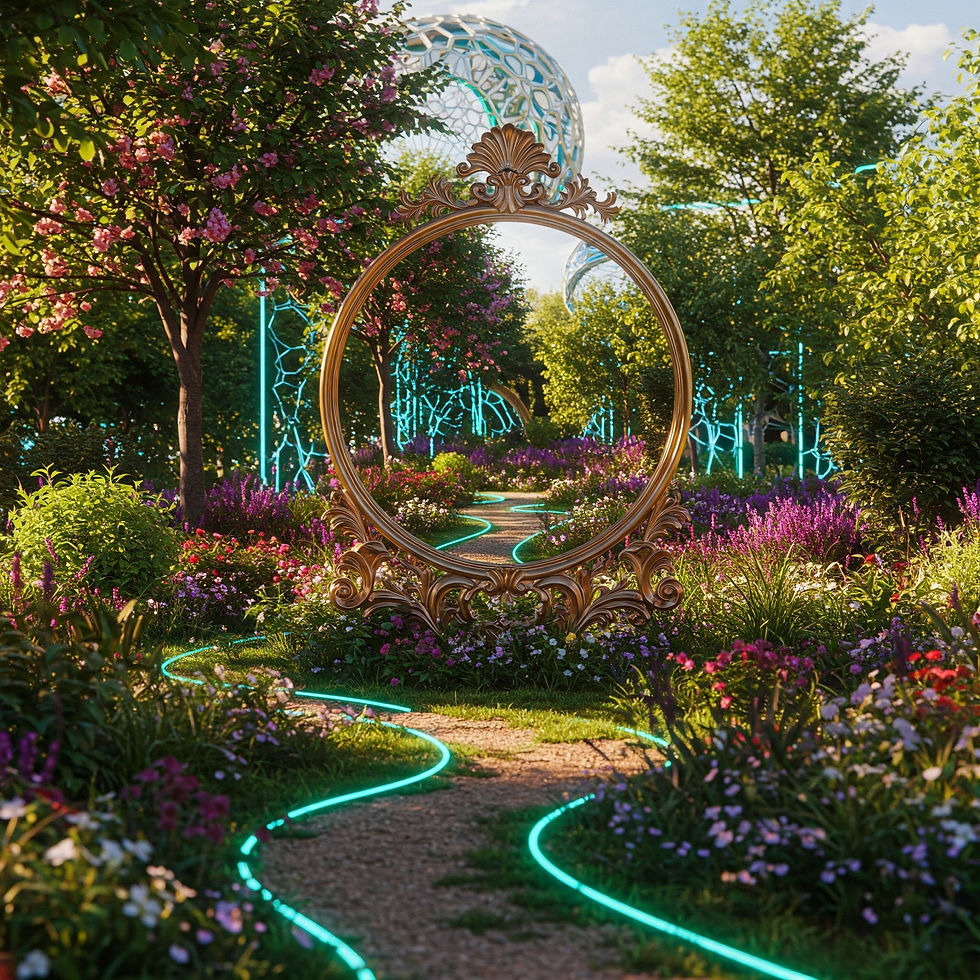
Artificial Intelligence (AI) is rapidly transforming our world, promising efficiency, objectivity, and data-driven insights. Yet, this promise is often undermined by the presence of cognitive biases within AI systems. These biases, mirroring our own human fallibilities, can lead to skewed judgments, unfair outcomes, and a perpetuation of societal inequalities. Understanding the intricacies of these biases, their origins, and their impact is crucial for developing responsible and ethical AI.
Deconstructing Cognitive Biases: More Than Just Errors
Cognitive biases are systematic deviations from rational judgment. They represent mental shortcuts our brains use to navigate a complex world, but these shortcuts can lead to consistent errors in thinking. In AI, these biases aren't simply random errors; they are patterned and predictable, often reflecting underlying societal biases present in the data. Let's delve deeper into some key examples:
Confirmation Bias: The tendency to seek and interpret information that confirms pre-existing beliefs while ignoring or downplaying contradictory evidence. In AI, this can manifest as the model prioritizing data that aligns with its initial assumptions or the biases of its creators, even if those assumptions are flawed. This can lead to a self-reinforcing cycle where the AI continuously validates its own flawed understanding.
Anchoring Bias: Over-reliance on the first piece of information received (the "anchor") when making decisions, even if that information is irrelevant. An AI might fixate on an initial data point, skewing its subsequent analysis and leading to inaccurate or biased conclusions. For example, in a pricing algorithm, an initial suggested price might unduly influence the final price, even if subsequent market data suggests a different value.
Availability Heuristic: Judging the likelihood of an event based on how easily examples come to mind. If an AI is trained on data that overrepresents certain events (e.g., due to media coverage or skewed data collection), it might overestimate their probability. This can lead to biased risk assessments, for example, in criminal justice or loan applications.
Groupthink Bias: The desire for harmony within a group can lead to suppressing dissenting opinions. In AI development, if the team lacks diversity or critical perspectives, biases in the model can go unchecked. This can result in AI systems that reflect the narrow worldview of their creators, perpetuating existing inequalities.
Automation Bias: The tendency to over-trust automated systems, even when they are wrong. Users might blindly accept an AI's output without questioning its validity, leading to potentially harmful consequences, especially in critical domains like healthcare or aviation.
Selection Bias: Occurs when the data used to train the AI is not representative of the population the AI is intended to serve. This can lead to biased predictions and unfair outcomes for underrepresented groups. For example, if a medical AI is trained primarily on data from men, it might be less accurate in diagnosing women.
The Genesis of Bias in AI: Data, Algorithms, and Humans
Bias in AI originates from multiple sources, primarily related to the data it learns from, the algorithms used, and even the humans involved in the development process:
Biased Training Data: This is the most significant source of bias. If the data used to train an AI model is not representative, the model will learn and perpetuate the biases present in the data. This can be due to historical biases, sampling bias, or simply a lack of diversity in the data.
Algorithmic Bias: Even with seemingly unbiased data, the algorithms themselves can introduce bias. Different algorithms have different strengths and weaknesses, and some might be inherently more susceptible to certain types of bias. For example, some algorithms might overfit the training data, leading to poor generalization on new, unseen data, particularly for underrepresented groups.
Feature Selection Bias: The process of choosing which features to include in the model can inadvertently introduce bias. If the selected features are correlated with sensitive attributes (like race or gender), the model can learn to discriminate, even if those attributes are not explicitly included. This is sometimes referred to as "proxy discrimination."
Human Interaction Bias: The way humans interact with AI systems can also introduce bias. For example, if users tend to provide more positive feedback for certain types of outputs, the AI might learn to favor those outputs, even if they are not objectively better. This can create a feedback loop, reinforcing existing biases. Furthermore, biases in the design and development process, introduced by the human creators, can be embedded in the AI system.
The Real-World Ramifications: From Micro to Macro
The consequences of biased AI can be far-reaching and deeply damaging, impacting individuals and society as a whole:
Discrimination in Hiring and Employment: AI-powered recruitment tools can perpetuate existing biases, leading to unfair hiring practices and limiting opportunities for certain demographics.
Unjust Criminal Justice Outcomes: Risk assessment algorithms used in the criminal justice system can amplify racial biases, leading to harsher sentences and disproportionate targeting of minority communities.
Unequal Access to Financial Services: AI-based loan approval systems can discriminate against certain demographics, denying them access to credit and perpetuating economic inequality.
Biased Healthcare Decisions: AI systems used in healthcare can make biased diagnoses or treatment recommendations, leading to disparities in healthcare outcomes.
Social and Political Polarization: AI-powered recommendation systems on social media platforms can create echo chambers, reinforcing existing biases and contributing to social and political polarization.
Towards Fair and Ethical AI: A Multifaceted Approach
Combating bias in AI requires a comprehensive and multi-faceted strategy:
Data Diversity and Augmentation: Collecting diverse and representative data is paramount. Data augmentation techniques can also be used to balance datasets and reduce bias, but care must be taken to avoid introducing new biases in the augmentation process.
Algorithmic Fairness Techniques: Developing algorithms that are explicitly designed to be fair and unbiased is essential. This involves research into fairness metrics (e.g., equal opportunity, equal outcome) and techniques like adversarial debiasing, which aims to train models that are robust to bias.
Explainable AI (XAI): Making AI decision-making processes more transparent and explainable can help identify and address biases. XAI techniques can provide insights into how the model arrived at a particular decision, making it easier to detect and correct biases.
Human Oversight and Auditing: Human oversight is crucial to ensure that AI systems are not making biased or discriminatory decisions. Regular audits and evaluations of AI systems are necessary to identify and correct biases. Furthermore, human review of AI-driven decisions, particularly in sensitive domains, can provide a safeguard against biased outcomes.
Interdisciplinary Collaboration: Addressing bias in AI requires collaboration between computer scientists, social scientists, ethicists, legal experts, and policymakers. This interdisciplinary approach is essential for understanding the social and ethical implications of AI and developing effective solutions.
Education and Awareness: Raising awareness about the potential for bias in AI is crucial. Educating developers, users, and the public about the risks and how to mitigate them is essential for fostering responsible AI development and deployment.
The Ongoing Quest for Unbiased AI
Building truly fair and unbiased AI systems is an ongoing quest. It requires continuous research, development of new techniques, and a commitment to ethical AI development practices. By acknowledging the potential for bias, understanding its origins, and taking proactive steps to mitigate it, we can harness the power of AI for good and ensure that it benefits all of humanity, not just a privileged few. The shadow of bias must be recognized and addressed if we are to realize the full potential of AI.
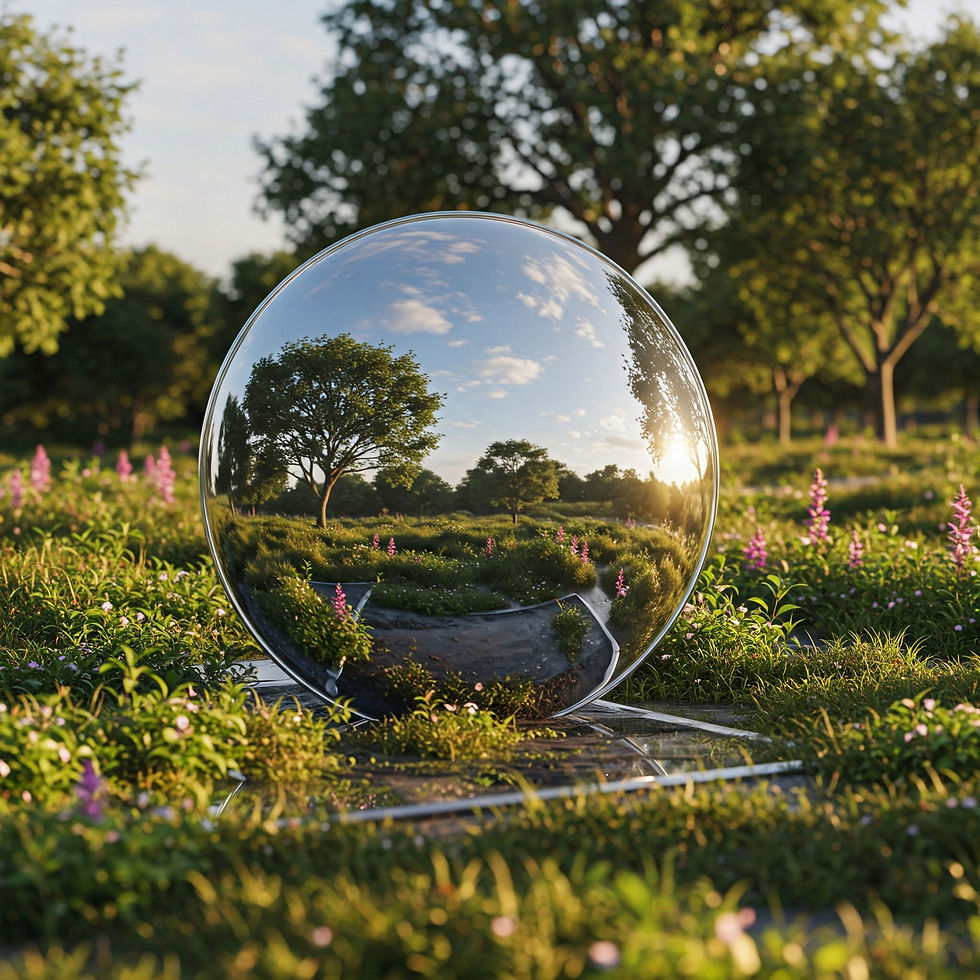
コメント