The AI Factory: Remodeling Manufacturing from the Ground Up
- Tretyak
- Mar 22
- 5 min read

1. How does AI improve predictive maintenance and reduce downtime?
Expanded Description:
AI-driven predictive maintenance transcends traditional time-based or reactive maintenance by employing sophisticated algorithms to anticipate equipment failures before they occur. This approach leverages the power of machine learning to analyze vast datasets of sensor readings, operational logs, and historical maintenance records, identifying subtle patterns and anomalies that indicate impending equipment malfunctions. By proactively addressing potential issues, manufacturers can minimize unplanned downtime, optimize maintenance schedules, and extend the lifespan of their valuable assets.
Expanded Detailed Functionality:
Advanced Sensor Fusion: Integrating data from diverse sensor types (vibration, temperature, acoustic, pressure, electrical current, etc.) to create a comprehensive picture of equipment health.
Complex Pattern Recognition: Utilizing deep learning models (e.g., Long Short-Term Memory (LSTM) networks, Convolutional Neural Networks (CNNs) for time-series data) to identify complex, non-linear relationships within sensor data.
Remaining Useful Life (RUL) Prediction: Employing survival analysis and regression models to estimate the remaining operational lifespan of equipment components.
Automated Root Cause Analysis: Using AI to analyze failure patterns and identify the underlying causes of equipment malfunctions, enabling targeted preventive measures.
Digital Twin Integration: Combining real-time sensor data with virtual replicas of equipment (digital twins) to simulate operating conditions and predict performance under various scenarios.
Reinforcement Learning for Maintenance Optimization: Using reinforcement learning to optimize maintenance schedules and policies based on real-time feedback and long-term cost considerations.
2. What are the applications of AI in quality control and defect detection?
Expanded Description:
AI-powered quality control systems revolutionize defect detection by automating visual inspection, analyzing complex material properties, and providing real-time feedback on production processes. These systems surpass human capabilities in speed, accuracy, and consistency, enabling manufacturers to achieve near-zero defect rates and enhance product quality.
Expanded Detailed Functionality:
3D Vision Systems: Employing 3D imaging and AI to detect defects in complex geometries and surface textures.
Thermal Imaging Analysis: Using infrared cameras and AI to identify thermal anomalies that indicate material defects or process irregularities.
X-ray and Ultrasound Analysis: Utilizing AI to analyze radiographic and ultrasonic images to detect internal defects in materials and components.
Automated Metrology: Integrating AI with precision measurement systems to automate dimensional inspection and ensure compliance with tolerances.
Generative Adversarial Networks (GANs) for Defect Simulation: Using GANs to generate realistic defect images for training AI models, improving defect detection accuracy.
Knowledge-Based Defect Classification: Developing AI systems that incorporate expert knowledge and domain-specific rules to classify and categorize defects.
3. How is AI being used to optimize supply chain management and logistics?
Expanded Description:
AI empowers manufacturers to build resilient and agile supply chains by providing advanced forecasting capabilities, optimizing inventory management, and streamlining logistics operations. By analyzing vast amounts of data from diverse sources, AI can anticipate demand fluctuations, identify potential disruptions, and optimize resource allocation.
Expanded Detailed Functionality:
Multi-Tier Supply Chain Visibility: Using AI to track materials and products across multiple tiers of the supply chain, providing end-to-end visibility.
Dynamic Pricing and Inventory Optimization: Employing AI to adjust pricing strategies and inventory levels in response to real-time market conditions.
AI-Powered Route Optimization: Utilizing AI to plan optimal transportation routes, considering factors such as traffic, weather, and delivery deadlines.
Blockchain Integration for Supply Chain Transparency: Combining AI with blockchain technology to ensure secure and transparent data sharing across the supply chain.
AI-Driven Supplier Risk Assessment: Using AI to evaluate supplier performance and identify potential risks, such as financial instability or capacity constraints.
Automated Warehouse Management: Implementing AI-powered robots and automated guided vehicles (AGVs) to optimize warehouse operations and improve efficiency.
4. What role does AI play in robotic automation and smart factories?
Expanded Description:
AI is the driving force behind the development of smart factories, where robots and machines can autonomously adapt to changing conditions, collaborate with humans, and optimize production processes in real-time. By integrating AI with robotics, sensor networks, and digital twins, manufacturers can create highly flexible and efficient production systems.
Expanded Detailed Functionality:
Human-Robot Collaboration (HRC): Developing AI algorithms that enable robots to understand human intentions and collaborate safely with workers.
Edge AI for Real-Time Control: Deploying AI algorithms at the edge of the network to enable real-time control of robots and machines.
AI-Powered Simulation and Optimization of Robotic Workcells: Using AI to simulate and optimize the performance of robotic workcells for specific tasks.
Distributed AI for Swarm Robotics: Developing AI algorithms that enable swarms of robots to coordinate their actions and perform complex tasks.
Digital Twin-Based Control: Using digital twins to simulate and control physical robots, enabling remote monitoring and management.
Adaptive Robotic Grasping: Employing AI to enable robots to grasp and manipulate objects with varying shapes and sizes.
5. How does AI enable personalized manufacturing and mass customization?
Expanded Description:
AI empowers manufacturers to meet the growing demand for personalized products by automating the design, production, and delivery of customized goods. By analyzing customer preferences and leveraging flexible manufacturing systems, AI enables mass customization at scale.
Expanded Detailed Functionality:
Natural Language Processing (NLP) for Customer Feedback Analysis: Using NLP to analyze customer reviews and feedback to identify product preferences and design requirements.
AI-Driven Product Configurators: Developing AI-powered tools that enable customers to design and customize products online.
On-Demand Manufacturing with 3D Printing: Using AI to optimize 3D printing processes for the production of customized parts and products.
AI-Powered Product Recommendation Systems: Employing AI to recommend personalized products based on customer browsing and purchase history.
Dynamic Production Scheduling for Customized Orders: Using AI to schedule production based on individual customer orders and delivery requirements.
AI for Modular Product Design: Designing products that are easily customizable and adaptable to individual customer needs.
6. How is AI used to improve energy efficiency and sustainability in manufacturing?
Expanded Description:
AI plays a crucial role in promoting sustainable manufacturing practices by optimizing energy consumption, reducing waste, and minimizing environmental impact. By analyzing real-time data from energy meters, production processes, and environmental sensors, AI can identify opportunities for improvement and implement energy-saving strategies.
Expanded Detailed Functionality:
AI-Driven Energy Management Systems: Employing AI to optimize energy usage in manufacturing facilities, considering factors such as production schedules, weather conditions, and energy prices.
AI for Predictive Maintenance of Energy-Intensive Equipment: Using AI to predict and prevent failures of energy-intensive equipment, such as furnaces and compressors.
AI-Powered Material Optimization: Employing AI to optimize material selection and usage, reducing waste and minimizing the environmental impact of production.
AI for Closed-Loop Recycling: Developing AI systems that optimize recycling processes and enable the recovery of valuable materials from waste streams.
AI-Driven Carbon Footprint Tracking and Reduction: Using AI to track and reduce the carbon footprint of manufacturing operations.
AI for Sustainable Supply Chain Management: Employing AI to optimize logistics and transportation, reducing fuel consumption and emissions.
7. What are the best AI powered tools for manufacturing?
Expanded Description:
The best AI-powered tools for manufacturing vary depending on the specific application and industry. However, some leading solutions include platforms for predictive maintenance, quality control, supply chain optimization, and robotic automation.
Expanded Examples:
C3 AI Reliability: A comprehensive AI platform for predictive maintenance and asset management.
Landing AI: A computer vision platform for automated visual inspection and quality control.
Element Analytics: A data analytics platform for industrial time series data.
Seebo: A process intelligence platform that uses AI to predict and prevent production losses.
Sight Machine: A manufacturing analytics platform that provides real-time insights into production performance.
UiPath: Robotic Process Automation (RPA) Platform with AI capabilities.
AWS IoT SiteWise: Cloud based platform for collecting, organizing, and visualizing data from industrial equipment.
Microsoft Azure IoT: Cloud based platform for creating IOT solutions, and using AI for predictive maintenence.
Google Cloud AI Platform: Cloud based platform for building, and deploying Machine learning models.
These expanded descriptions and functionalities highlight the transformative potential of AI in manufacturing and industry, showcasing how these technologies are enabling manufacturers to achieve unprecedented levels of efficiency, quality, and sustainability.
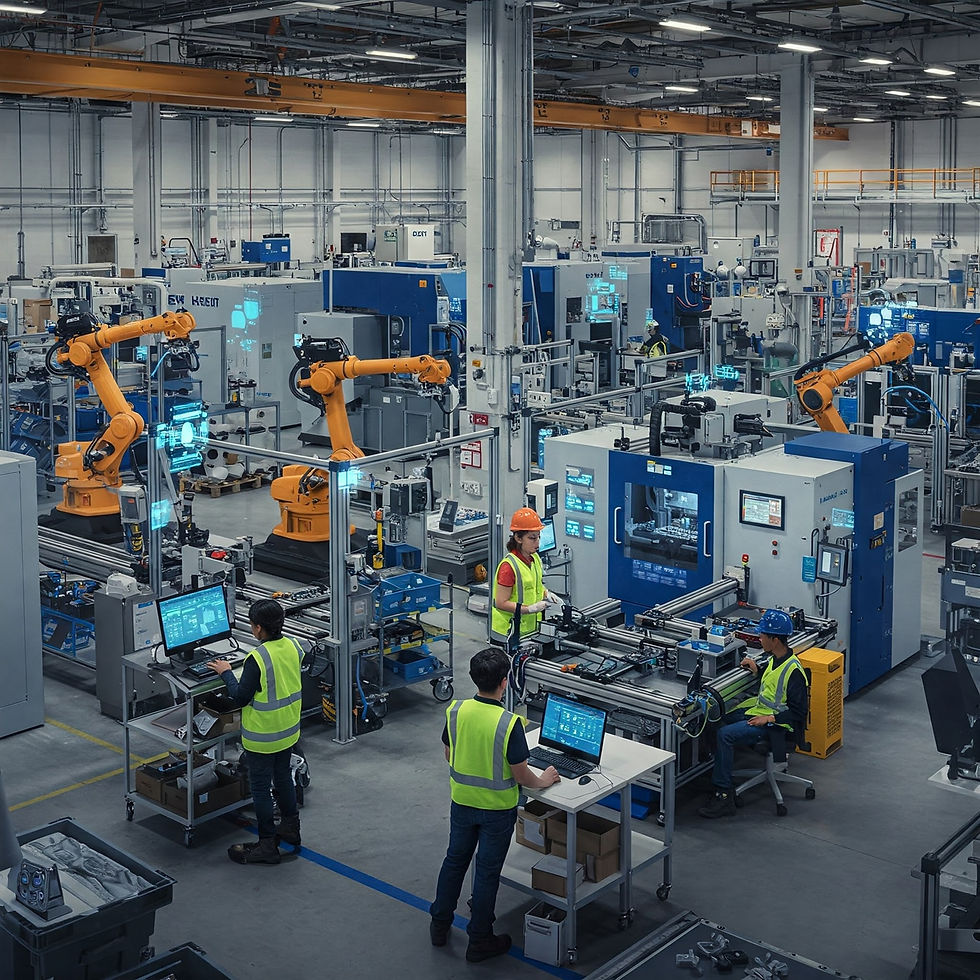
Comentarios