The AI Oracle: Unraveling the Enigma of AI Decision-Making
- Tretyak
- Feb 22
- 5 min read
Updated: Feb 28
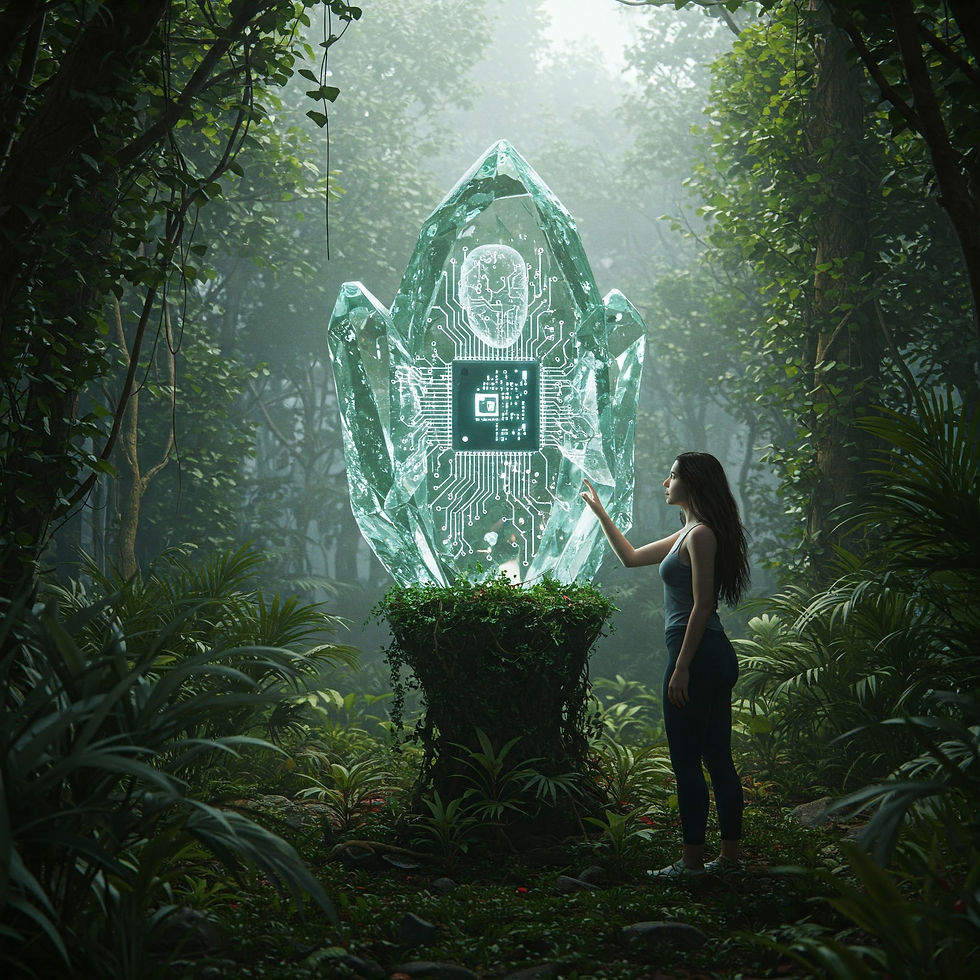
Artificial Intelligence is rapidly evolving into a ubiquitous decision-maker, shaping our world in ways both subtle and profound. From personalized recommendations to critical judgments in healthcare and finance, AI systems are entrusted with making choices that have far-reaching consequences. But how do these digital minds arrive at their conclusions? What intricate processes and factors guide their decision-making? And can we, as humans, truly understand and trust the reasoning behind their choices? This exploration delves deeper into the fascinating world of AI decision-making, uncovering the mechanisms, influences, and challenges that shape its trajectory.
Deconstructing the Decision-Making Engine: A Symphony of Algorithms and Data
At the heart of AI's decision-making prowess lies a powerful synergy between algorithms and data. Algorithms, the intricate sets of rules and instructions that govern the AI's thinking process, can range from simple "if-then" statements to complex neural networks with millions of interconnected nodes. These algorithms act as the scaffolding upon which AI's cognitive abilities are built.
Data, the lifeblood of AI, fuels these algorithms. Vast quantities of data are fed into the AI system, enabling it to discern patterns, identify trends, and make predictions. The more data it consumes, the more refined its decision-making becomes, allowing it to navigate complex scenarios with increasing accuracy.
Here's a more detailed breakdown of the typical AI decision-making process:
Data Acquisition and Preprocessing: The AI system first gathers data relevant to the decision it needs to make. This data can originate from various sources, including sensors, databases, images, text, and even social media feeds. The raw data is then preprocessed, cleaned, and transformed into a format suitable for the AI algorithms.
Feature Extraction and Engineering: The AI system identifies and extracts relevant features from the data. These features are the essential characteristics or attributes that the AI will use to make its decision. Feature engineering involves selecting, transforming, and creating features that best represent the underlying patterns in the data.
Model Training and Selection: The AI system uses the processed data and extracted features to train a machine learning model. This involves selecting an appropriate algorithm and adjusting its parameters to optimize its performance on the given data. The model learns from the data, identifying patterns and relationships that can be used to make predictions or decisions.
Prediction and Evaluation: Once the model is trained, it can be used to make predictions or evaluate different options. For example, it might predict the likelihood of a customer clicking on an ad or evaluate the potential risks and rewards of a financial investment.
Decision Optimization and Selection: The AI system uses its predictions and evaluations to select the best course of action. This decision is often guided by a predefined objective function, which specifies the goal the AI is trying to achieve. The AI might use optimization techniques to find the decision that maximizes the objective function.
Action and Feedback: The AI system takes action based on its decision. This action could be anything from recommending a product to a customer to controlling a robot in a factory. The system then gathers feedback on the outcome of its decision, which can be used to further refine its decision-making process.
The Puppeteers of Choice: Unraveling the Influences on AI Decisions
While AI's decision-making process might appear objective and purely data-driven, various factors can subtly or overtly influence its choices:
Data Bias: Biases present in the training data can significantly skew the AI's decisions. If the data is not representative of the real world or reflects historical or societal biases, the AI's decisions will likely perpetuate those biases. For example, a loan approval system trained on historical data that reflects past discrimination might unfairly deny loans to certain demographics.
Algorithmic Bias: Even with unbiased data, the algorithms themselves can introduce bias. Different algorithms have different strengths and weaknesses, and some might be inherently more prone to certain types of bias. For example, some algorithms might overfit the training data, leading to poor generalization and biased predictions on new, unseen data.
Objective Function: The objective function, which defines the goal the AI is trying to achieve, can significantly shape its decisions. For example, an AI tasked with maximizing advertising revenue might prioritize click-through rates over the quality or relevance of the content.
Human Input: Human input can influence AI decisions in various ways. For example, a doctor might override an AI's diagnosis based on their own clinical judgment, or a user might provide feedback that influences the AI's learning process.
Explainability and Interpretability: The ability to understand and interpret the AI's decision-making process is crucial for building trust and ensuring responsible use. Explainable AI (XAI) techniques aim to make AI's reasoning more transparent, allowing humans to understand why the AI made a particular decision.
Lifting the Veil: Explainable AI and the Quest for Transparency
One of the significant challenges with AI decision-making is the "black box" problem. Many AI systems, particularly those based on deep learning, arrive at their conclusions through complex computations that are not easily understandable to humans. This lack of transparency can make it difficult to trust AI's decisions, especially in high-stakes domains like healthcare and finance.
Explainable AI (XAI) aims to address this challenge by making AI's decision-making processes more transparent and interpretable. XAI techniques can provide insights into how the AI arrived at a particular decision, helping humans understand the factors that influenced its choice.
Some common XAI techniques include:
Decision Trees: Visualizing the AI's decision-making process as a tree-like structure, showing the different factors and criteria that led to the final decision.
Rule Extraction: Extracting a set of rules that the AI is following, making its decision-making process more explicit and understandable.
Saliency Maps: Highlighting the parts of the input data that were most influential in the AI's decision, helping humans understand which factors were most important.
Counterfactual Explanations: Generating explanations by showing how the AI's decision would have changed if the input data were different. This helps humans understand the sensitivity of the AI's decision to different factors.
Explainable AI is crucial for building trust in AI systems, ensuring that they are used responsibly and ethically, and enabling humans to understand and potentially correct biases or errors in the AI's decision-making process.
The Evolving Landscape of AI Decision-Making
The field of AI decision-making is constantly evolving, with new techniques and approaches emerging rapidly. As AI systems become more sophisticated and are deployed in increasingly complex and critical domains, the need for transparency, explainability, and ethical considerations will only grow.
The future of AI decision-making will likely involve:
More sophisticated XAI techniques: Enabling deeper understanding of AI's reasoning processes.
Human-AI collaboration: Combining the strengths of human and artificial intelligence to make better decisions.
Ethical frameworks and regulations: Guiding the development and deployment of AI systems to ensure fairness, accountability, and transparency.
As AI continues to shape our world, it's essential to engage in ongoing dialogue and collaboration between AI researchers, ethicists, policymakers, and the public to ensure that AI decision-making aligns with human values and serves the betterment of society.
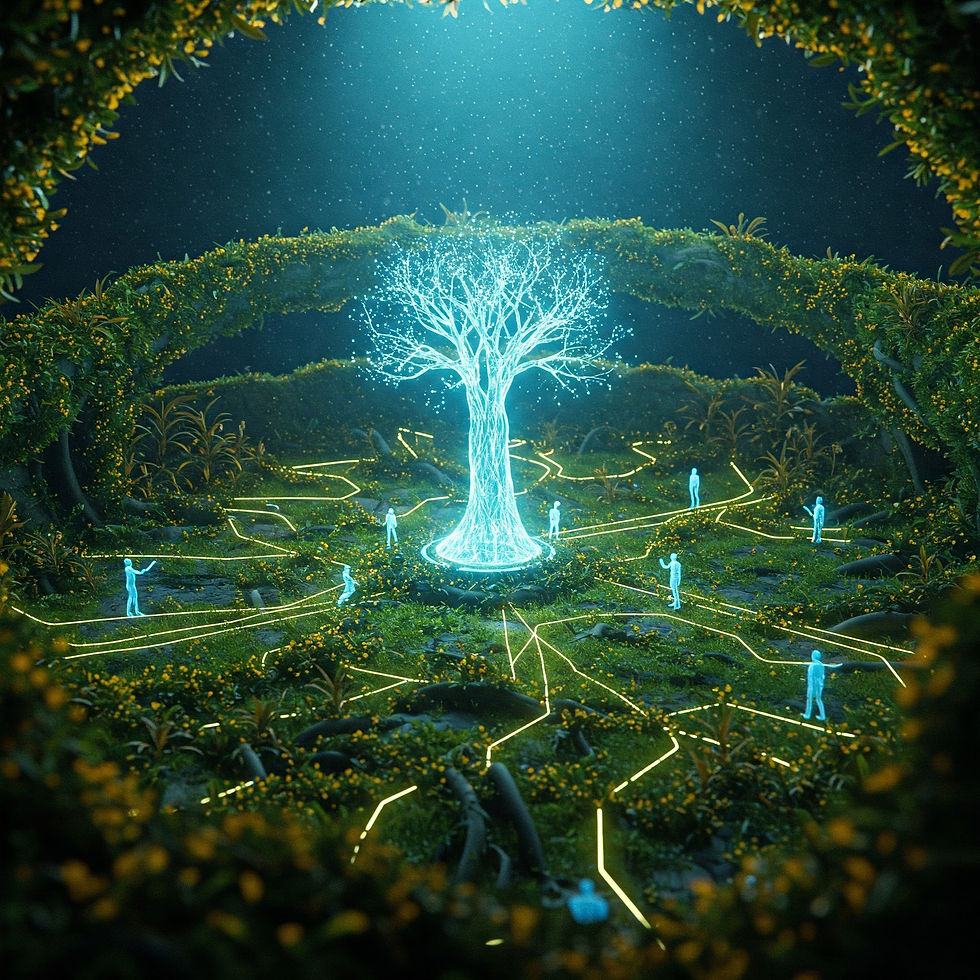
Comments