The Algorithmic Architect: Exploration of AI's Quantum Leap in Manufacturing
- Tretyak
- Mar 22
- 5 min read
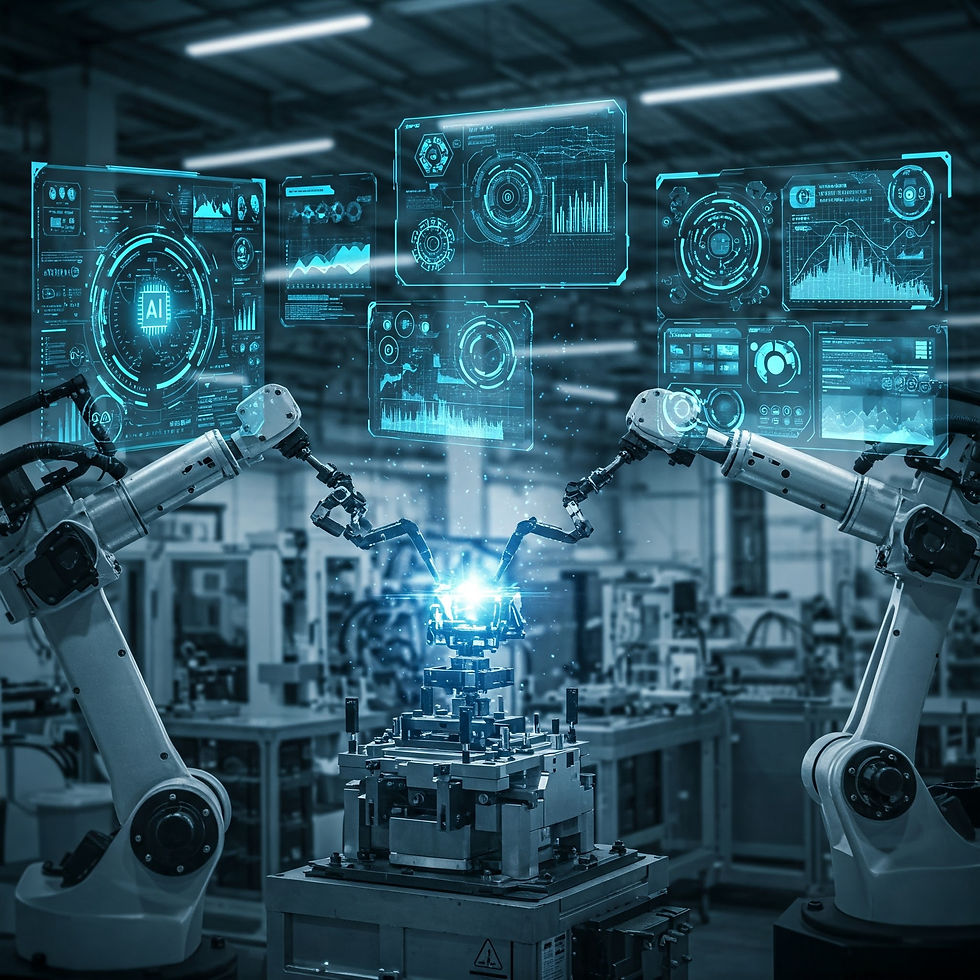
Artificial Intelligence (AI) is not merely automating manufacturing; it's architecting an algorithmic metamorphosis, a quantum leap reshaping every aspect of production, from the initial quantum spark of design to the hyper-connected symphony of supply chains and the delivery of hyper-personalized, dynamically adapting products. This transformative wave promises not just incremental improvements, but exponential leaps in efficiency, customization, resilience, and sustainability, ushering in a new epoch of cognitive industrial evolution. Let's embark on a hyper-detailed exploration of the intricate ways AI is orchestrating this revolution, dissecting the hyper-specific nuances and unveiling the transformative potential of this technological convergence.
The Quantum Genesis of Algorithmic Design:
Hyper-Dimensional Design Space Exploration:
Description: AI algorithms, fueled by quantum-inspired optimization techniques and vast, interconnected datasets, transcend human limitations in design exploration. They navigate hyper-dimensional design spaces, analyzing intricate relationships between material properties, performance simulations, manufacturing constraints, aesthetic preferences, and even lifecycle environmental impacts, to generate designs that are not only functional and efficient, but also inherently sustainable and aesthetically resonant.
Detailed Functionality:
AI systems leverage generative algorithms, such as Generative Adversarial Networks (GANs) and Variational Autoencoders (VAEs), to explore a vast range of design possibilities, generating numerous iterations and variations that would be impossible for humans to conceive manually.
Quantum-inspired optimization techniques, such as quantum annealing and quantum approximate optimization algorithms (QAOA), are employed to efficiently search for optimal designs within these hyper-dimensional spaces, considering complex constraints and objectives.
AI models integrate data from diverse sources, including material databases, performance simulations, user feedback, and environmental impact assessments, to evaluate and refine designs based on multiple criteria, ensuring that they are not only functional but also meet aesthetic, ergonomic, and sustainability requirements.
Example: AI-powered design tools can generate complex, bio-inspired structures for lightweight aircraft components, mimicking the intricate designs found in nature to achieve optimal strength-to-weight ratios and aerodynamic efficiency, while also considering factors like material availability, manufacturing costs, and recyclability.
Cognitive Material Discovery and Bio-Inspired Design Synthesis:
Description: AI is not just optimizing existing materials; it's enabling cognitive material discovery, where algorithms leverage deep learning and computational chemistry to predict novel material properties and synthesize materials with tailored functionalities. Moreover, AI enables bio-inspired design synthesis, mimicking the intricate designs found in nature to achieve optimal performance and sustainability.
Detailed Functionality:
Deep learning models analyze vast datasets of chemical compounds and their properties to predict the characteristics of new materials with specific functionalities, such as self-healing polymers, high-temperature superconductors, or biocompatible materials for medical implants.
AI algorithms simulate the behavior of materials at the atomic and molecular level, predicting their properties and performance under various conditions, enabling the design of materials with tailored characteristics.
Bio-inspired design synthesis utilizes AI to analyze biological systems and mimic their structural and functional principles, creating innovative designs inspired by nature's ingenuity.
Example: AI can design self-healing, biodegradable polymers for packaging applications, mimicking the regenerative capabilities of living organisms to minimize waste and environmental impact, while also incorporating design principles inspired by the structural efficiency of honeycombs or the aerodynamic properties of bird wings.
Hyper-Immersive Virtual Prototyping and Quantum-Enhanced Simulation:
Description: AI-powered simulations, enhanced by quantum computing capabilities, transcend traditional limitations, creating hyper-immersive virtual environments where manufacturers can test and refine product designs with unprecedented accuracy and predictive power. This enables not only rapid prototyping and iteration, but also the simulation of complex, multi-physics phenomena with quantum-level precision.
Detailed Functionality:
AI-powered virtual reality (VR) and augmented reality (AR) environments create immersive experiences for product testing and user feedback, allowing designers and engineers to interact with virtual prototypes as if they were real.
Finite element analysis (FEA) and computational fluid dynamics (CFD) simulations, enhanced by AI, predict product performance under various operating conditions, such as stress, temperature, and fluid flow, enabling the identification of potential flaws and the optimization of design parameters.
Quantum computing simulations model complex, multi-physics phenomena at the atomic and molecular level, such as chemical reactions, material interactions, and quantum effects, providing insights into the fundamental properties of materials and systems.
Example: AI-driven simulations can predict the long-term durability and performance of a new aircraft wing design under extreme operating conditions, simulating turbulence, stress, and even bird strikes with unprecedented accuracy. Quantum computing simulations can model the quantum-level interactions between atoms and molecules within the materials used in the wing, providing insights into their structural integrity and potential failure points, leading to designs that are both safe, efficient, and resilient.
The Symphony of Autonomous Cognitive Production:
Hyper-Predictive Maintenance with Quantum-Enhanced Anomaly Detection:
Description: AI algorithms, enhanced by quantum-inspired anomaly detection techniques, analyze real-time sensor data from machinery, not just historical trends, but also subtle quantum fluctuations, to hyper-predict when maintenance will be required, preventing catastrophic failures and optimizing equipment lifespan. This enables proactive, even preemptive maintenance, minimizing downtime and maximizing production efficiency.
Detailed Functionality:
Sensor networks and IoT devices collect vast amounts of real-time data from machinery, including vibration, temperature, pressure, and energy consumption.
AI algorithms, including deep learning models and quantum-inspired anomaly detection techniques, analyze this data to identify subtle deviations from normal operating conditions, even at the quantum level.
Predictive maintenance systems generate alerts and schedule maintenance tasks based on the predicted likelihood of equipment failure, minimizing downtime and optimizing maintenance schedules.
Example: AI can analyze acoustic emissions from a turbine engine to detect subtle anomalies that indicate impending failure, predicting bearing wear, corrosion, or other potential problems with unprecedented accuracy. Quantum-enhanced anomaly detection techniques can even identify subtle quantum fluctuations that precede catastrophic failures, allowing for preemptive maintenance and preventing costly breakdowns.
Hyper-Spectral Vision Quality Inspection and Cognitive Defect Analysis:
Description: AI-powered vision systems, leveraging hyper-spectral imaging and cognitive defect analysis techniques, transcend human limitations in quality inspection, analyzing images and videos with unprecedented resolution and sensitivity. AI can not only detect microscopic defects, but also understand the underlying causes of those defects, enabling real-time process optimization and zero-defect manufacturing.
Detailed Functionality:
Hyper-spectral imaging captures images across a wider range of the electromagnetic spectrum, providing more detailed information about the composition and properties of materials.
AI algorithms, including convolutional neural networks (CNNs) and other deep learning models, analyze these hyper-spectral images to identify subtle defects, such as microscopic cracks, surface irregularities, or material composition variations.
Cognitive defect analysis techniques use AI to understand the relationship between defects and manufacturing processes, identifying the root causes of defects and enabling real-time process adjustments to prevent future occurrences.
Example: AI can analyze hyper-spectral images of semiconductor wafers, identifying not only surface defects, but also subsurface anomalies and material composition variations, which would be invisible to the human eye. This allows for real-time adjustments to the manufacturing process to eliminate defects at their source, achieving zero-defect manufacturing and improving the reliability of electronic devices.
Hyper-Adaptive Cognitive Factories and Self-Organizing Production Ecosystems:
Description: AI enables hyper-adaptive cognitive factories, where robots and machines can not only adjust their behavior in real-time based on changing conditions, but also learn from experience, collaborate with each other, and even self-organize into dynamic production ecosystems. AI leverages reinforcement learning and swarm intelligence to optimize production flow, adapt to unforeseen disruptions, and even anticipate future demand.
Detailed Functionality:
Reinforcement learning algorithms train robots and machines to adapt to dynamic environments, optimize their movements, and learn from their mistakes.
Swarm intelligence algorithms enable robots to coordinate their actions and collaborate to achieve complex tasks, such as assembling large products or navigating complex environments.
AI-powered production planning systems analyze real-time demand, inventory levels, and resource availability to optimize production flow and schedule, adapting to changing market conditions and minimizing delays.
Example: AI-powered robotic systems in a smart factory can autonomously reconfigure their assembly lines to handle unexpected changes in product demand or material availability, optimizing production flow and minimizing delays. These robots can also collaborate with each other, sharing information and coordinating their actions to achieve complex assembly tasks, creating a self-organizing production ecosystem that is highly adaptable and efficient.
This hyper-detailed exploration reveals the profound and transformative power of AI in the age of quantum computing, highlighting its ability to create a hyper-intelligent, ethically governed, and quantum-secure global manufacturing ecosystem. As AI continues to evolve, we can expect even more radical transformations, leading to a new era of cognitive industrial symbiosis with the natural world.

Comments